Data labeling is a critical step in training AI models, as it directly influences their accuracy and performance. Properly labeled data provides the necessary context and information for algorithms to make informed decisions.
In this blog post, we’ll talk about the significant impact of data labeling on AI model accuracy and why businesses should prioritize high-quality labeling to achieve optimal results.
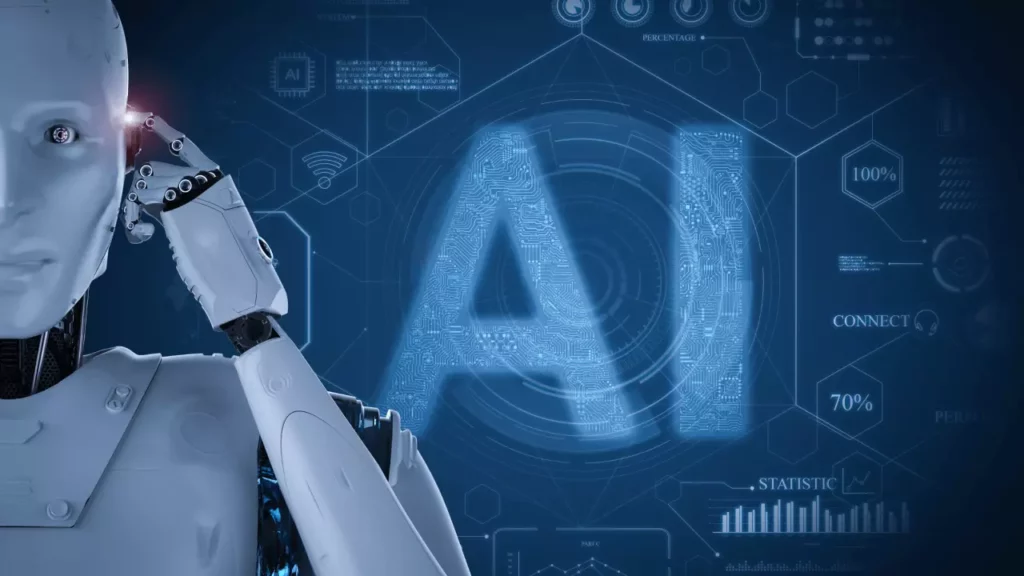
1. The Foundation of AI: Quality Data Labeling
Data labeling forms the bedrock on which AI models are built. Without accurate and consistent annotations, models may struggle to comprehend the intricacies of the data they are processing.
Flawed or insufficient labeling can lead to biased models, poor generalization, and inaccurate predictions, hampering the AI system’s effectiveness.
2. Garbage In, Garbage Out: The Importance of Accurate Labels
The phrase “garbage in, garbage out” applies aptly to AI model training. When faulty data labels are used during training, the model’s output will be equally unreliable.
Even the most sophisticated algorithms will struggle to produce accurate results if the labeled data fed into them is of poor quality.
3. The Labeling Bottleneck: Time and Resources
Data labeling can be a time-consuming and resource-intensive process. Manual labeling tasks, especially on large datasets, can slow down AI development significantly.
Investing in efficient data labeling tools and strategies can speed up the process, reducing the labeling bottleneck and accelerating AI model deployment.
4. Data Labeling Bias: Impact on Model Fairness
Bias in data labeling can result in biased AI models. If the training data is not representative of the real-world scenarios the model will encounter, it may produce discriminatory or unfair outcomes.
Avoiding bias requires careful curation of training data and implementing rigorous quality control measures during labeling.
5. Active Learning: Maximizing Labeling Efficiency
Active learning can enhance efficiency by selecting the most informative samples for annotation.
This approach reduces the labeling workload while ensuring the model focuses on crucial data points for improved accuracy. Incorporating active learning techniques can lead to significant savings in time and resources.
6. Scaling AI: Challenges of Large-Scale Data Labeling
As AI applications become more ambitious and widespread, scaling becomes a significant challenge.
Businesses must find ways to label large volumes of data accurately and swiftly. Embracing crowdsourcing platforms and leveraging pre-trained models can ease the burden of scaling efforts.
Conclusion
The accuracy of AI models is directly linked to the quality of data labeling they receive. Flawed labels can lead to unreliable predictions, biased outcomes, and diminished trust in AI systems.
Businesses must recognize the importance of data labeling in the AI development process and invest in robust strategies, tools, and techniques to ensure accurate, unbiased, and efficient labeling.
By prioritizing data labeling excellence, companies can unlock the full potential of AI and achieve superior results in their AI-driven applications.
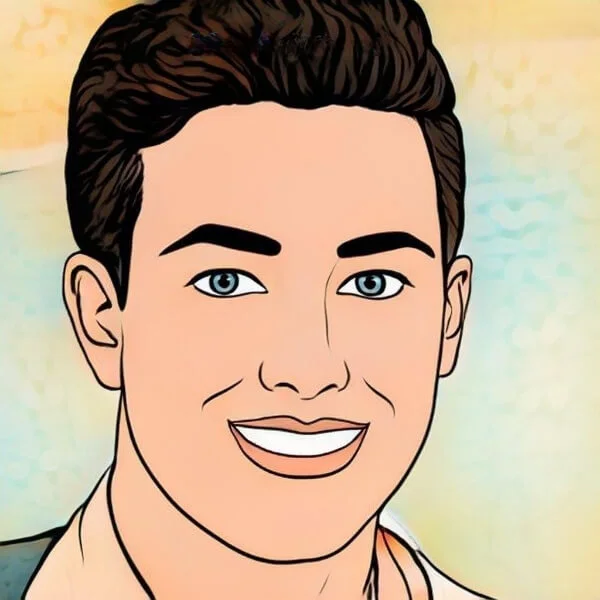
Glen Macey is an expert in all things technology. He enjoys writing blogs about how to use the latest software and hardware on the market, while also providing advice for using existing technology more effectively. His favorite pastimes include reading comic books and playing video games.